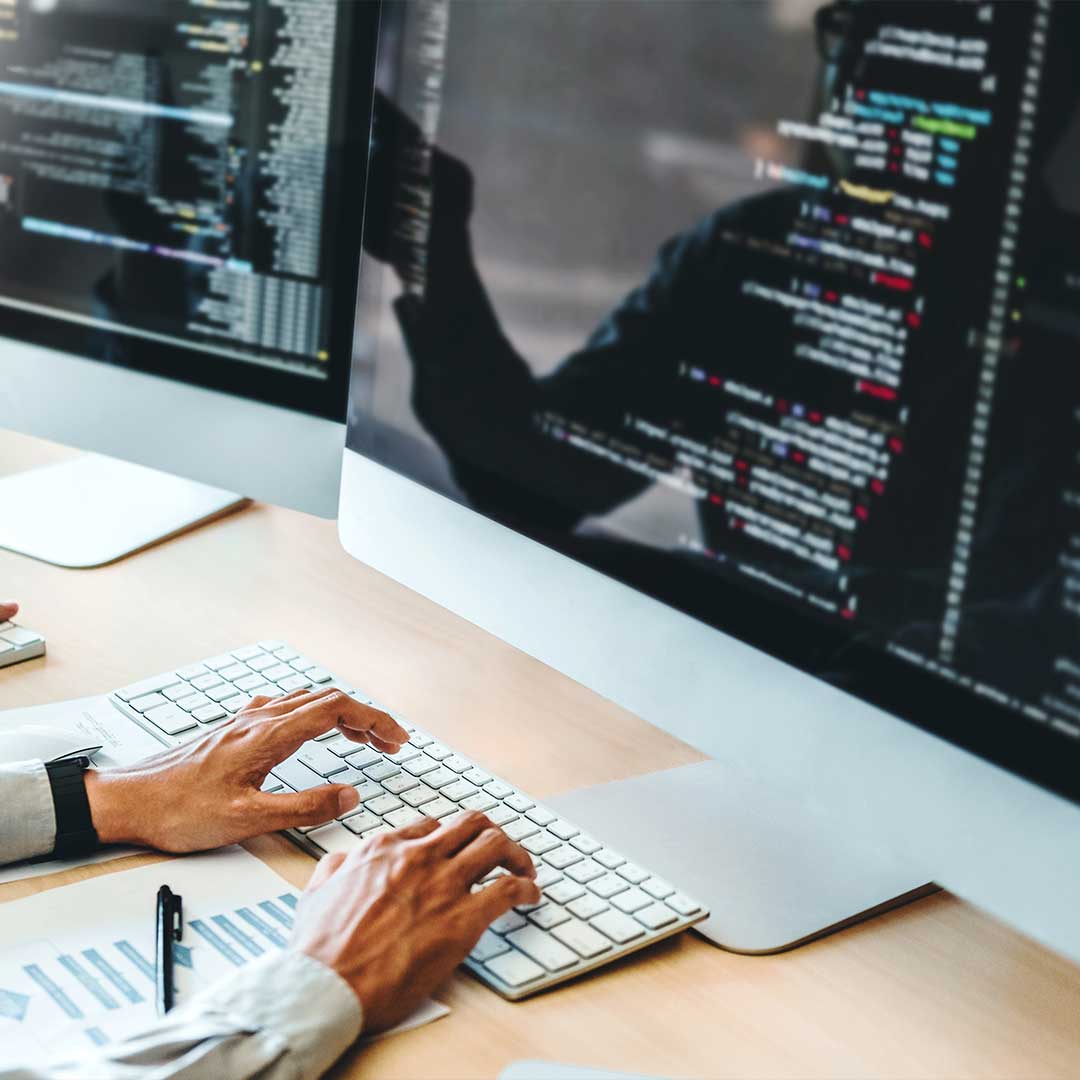
Integration Options
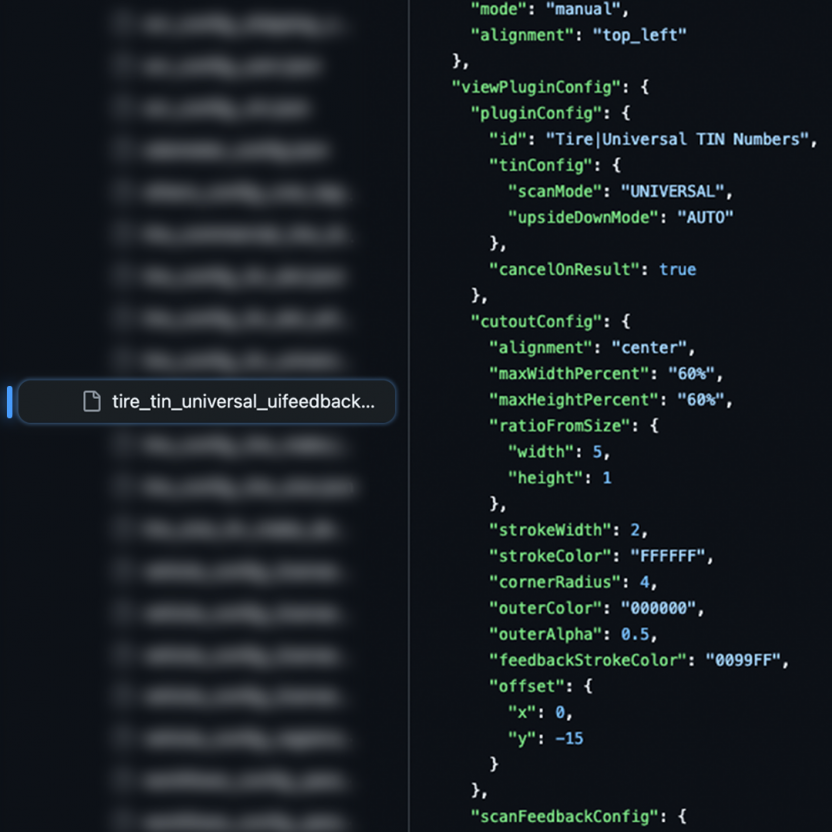
Test, Build, and Launch New Solutions Quickly
Access over 100 config files via our developer repositories. These config files include pre-defined example codes that serve as your blueprint for development, streamlining the process of testing, building, and launching new apps or web apps.
Access Developer Repositories

Create a Unique Scan Experience
Anyline enables you to create a fully tailored scan experience for your users. Write JSON strings that adjust guidance features like real-time visual, haptic, or audio feedback, overlays, scan cutouts, front or back camera activation, zoom gestures, flashlight activation, and more to cater to the unique needs of your users. This further enhances the scanning experience and ensures that users can successfully capture data from the target object.
Learn more about guidance features
Get Started in 3 Simple Steps
Step 1
Identify the platform you are developing on (Learn more by looking at our documentation)
Anyline Documentation
Step 3
Access the Developer Repository for your chosen platform and start testing!
Developer Repositories