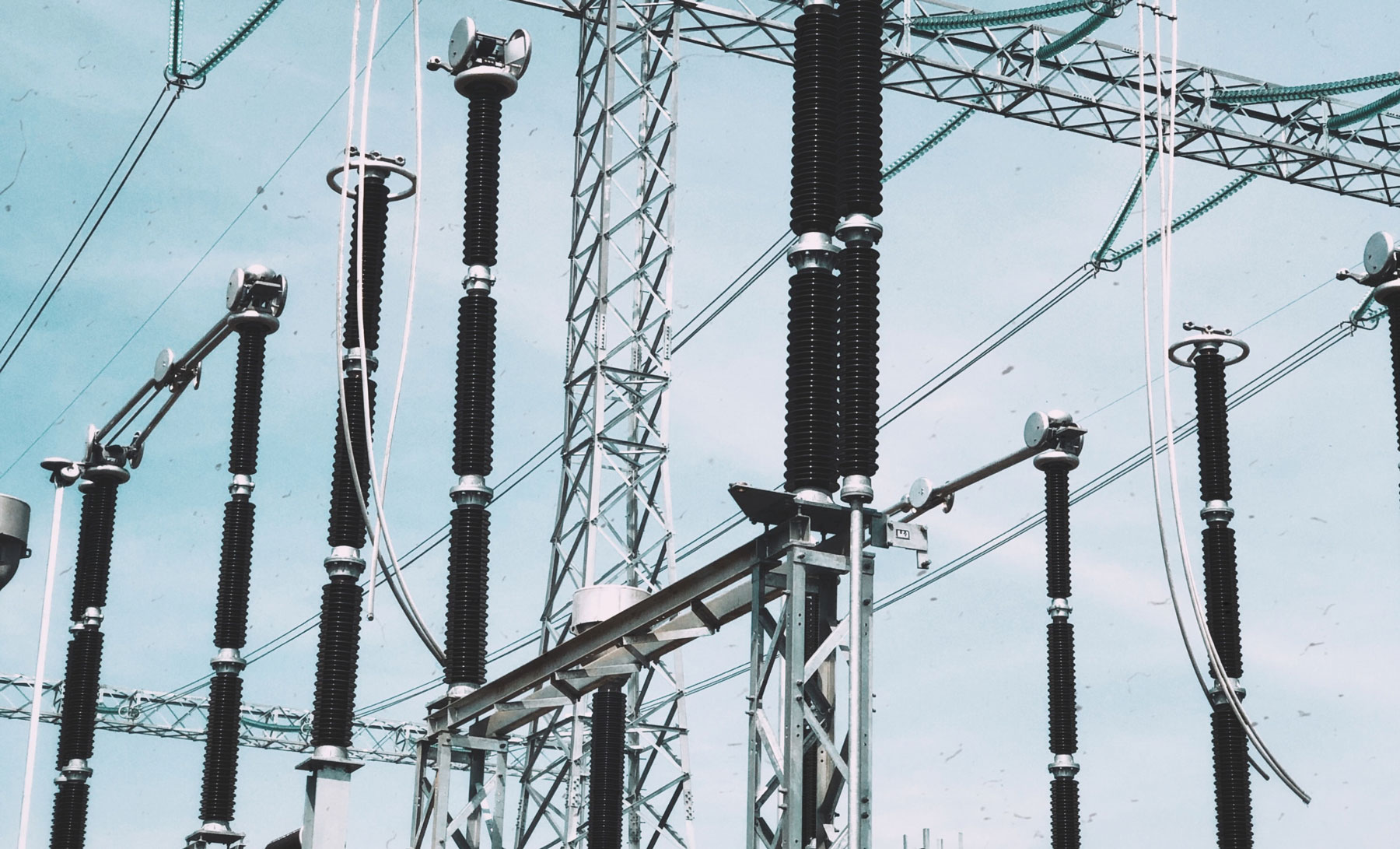
How to Detect Non-Technical Losses in Energy and Utility Operations
Electrical energy losses in power grids at the transmission and distribution level can be separated into technical losses (TLs) and non-technical losses (NTLs). Technical losses are unavoidable, and are the result of failures or issues with equipment and infrastructure during the transmission and distribution process. By contrast. non-technical losses occur due to a variety of controllable reasons. The most common causes of NTLs include non-billed or incorrectly billed electricity, as well as illegal or fraudulent use of electricity.
Fraudulent actions by energy customers are the result of everyday fraud, regularized corruption, and organized crime. But while it takes place more than one may want to believe, non-technical losses are the easiest losses for utilities to control and reduce. In this blog, we will outline how utility and energy providers can properly identify NTLs so that they can be reduced, and revenue streams can become more resilient.
1. Methods for NTL detection
The feature-based NTL detection technique is one of the most important and accessible ways for NTL identification when using machine learning algorithms – the most common approach that is in practice today.
In this approach, energy-use data is examined and a number of key data points are extracted which represent the most relevant aspects of each consumer. Using this information, customers are then classified as honest or fraudulent based on the analysis of the results.
A few specific examples of the most common features used in determining the honesty of the customer include:
- Max/Min, Standard Deviation, Average, Monthly kWh consumption – Energy consumption feature for a given period.
- Load – The ratio of average load to the peak load over a given period.
- Estimated readings – The number of estimated readings that are charged by the power distribution companies because of their inability to obtain the actual reading.
- Reduction in energy consumption – The decrease in energy usage during a specified period compared to the previous reading of the same duration.
- Seasonal consumption difference – Total energy usage by the customer in the particular season compared to the energy consumption of another season.
- Predicted kWh – The difference between the observed active energy consumption value to the anticipated value.
By collecting and comparing the various features, you can more accurately determine if there are any NTLs taking place, as well as where you can identify them to remove or reduce their impact on the energy consumption losses.
2. Performance Metrics Used for NTL Detection
Of all the performance metrics used for NTL detection, two appear more often than any other. Accuracy and detection rate are the most widely utilized matrix models used for NTL detection and in nearly all data-based methods.
Simply put, a higher detection rate and accuracy indicate that the model is well-functioning and has strong classification abilities for samples from both groups. The stronger your detection and accuracy rate, the better equipped you are for detecting NTLs on a regular basis.
While these two common performance metrics are the core of most NTL detections, operators need to consider a few others where the typical data sets for both honest and fraudulent are imbalanced. To ensure optimum accuracy with performance metrics, equal data sample sizes must be obtained.